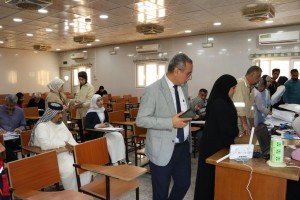
The College of Education for Pure Sciences discussed a master's thesis on
(Diffusion Models for Image Synthesis)
The thesis submitted by the researcher (Sara Ahmed Abdel Nema)
in the field of recent developments in medical imaging as well as the importance of creating high-quality synthetic images to train and test diagnostic algorithms without compromising patient privacy.
The study dealt with a comprehensive model that uses diffusion denoising probabilistic models (DDPM) to generate synthetic images for three types of medical images: magnetic resonance imaging (MRI), computed tomography (CT), and ultrasound images. Three separate DDPM models were developed and trained, with a focus on synthesizing images that simulate realistic medical scenarios.
The thesis concluded that the models achieved high accuracy in distinguishing between normal and abnormal images, achieving an accuracy of up to 98.06% for CT, 96.97% for MRI, and 97.47% for ultrasound images. The model also achieved high performance metrics including: MSE 0.0002, PSNR 43.1588, SSIM 0.9923, and F1 0.9733. The thesis aims that DDPM models are capable of producing accurate and realistic medical images that can be used in educational and clinical settings, while enhancing the accuracy and reliability of automated diagnostic tools, which supports the possibility of improving healthcare systems.