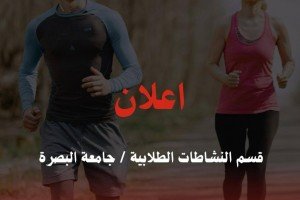
The College of Education for Pure Sciences at the University of Basrah discussed a PhD thesis on neural networks for some Bernstein sequences
The thesis presented by the researcher (Ibtihal Jassim Muhammad) included a set of new neural networks and knowledge using improved polynomials of the Bernistan polynomials, which relied on a positive integer coefficient.
Neural networks have been defined and studied using (Bernstan quadratic polynomials and Semi-Bernstan polynomials). Then the new resulting networks were improved by adding some parameters to the function processed by the network in more than one way. The previous networks were generalized by defining the generalizations of the Princetan polynomials, where they were known (Bernstan polynomials with integer powers and partial Princestan polynomials). Approximation theorems were presented and demonstrated for all networks defined in function space with continuous and restricted real values and with more than one dimension. As well as studying the approximation orders of these networks using the absolute moment and the functions belonging to the space of Labels. Numerical examples were given to demonstrate the efficiency of these networks and to calculate the error function and two test functions by selecting different values of the network parameters.
Thesis aims
Defining neural networks that are more efficient than the known neural networks in this field.
Thesis concluded
To prove that the new neural networks have a better approximation than the previous networks, both theoretical and numerical. Especially when increasing the values of the parameters in them, which leads to saving the effort resulting from the large number of arithmetic operations of the old networks, as well as the increase in the accuracy of the improved networks.
Dissertation recommended
To the use of new neural networks in applied fields because of their great ability in the speed and accuracy of approximation compared to similar ones known in this field.