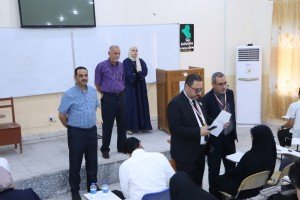
The College of Education for Pure Sciences at the University of Basrah discussed a master’s thesis on expanding the X-ray database for diagnosing corona virus using Conditioned Generative Conflict Networks (CGAN)
The message presented by the researcher (Haneen Majid Muhammad) included
Deep learning models have significantly and effectively excelled in many applications of computer vision in the medical fields. Despite this rapid superiority, deep learning models require large amounts of data in order for the training to be successful and with accurate results. Medical images lack the availability of large data sets due to the privacy of patients, so it is difficult to access this data compared to other categories, and adding notes to medical images is costly and time-consuming, one of the newly spread chest diseases is the Corona virus (COVID-19) . The chest images for this disease are very few, and the process of obtaining and collecting them is difficult and dangerous, and therefore we need a safe way to collect these images. To solve this problem, we proposed Conditional Generative Conflict Networks (CGAN) as a solution to increase the number of chest images data by generating artificial (pseudo) images, after generating a large number of data a deep learning model is designed to diagnose COVID-19 within an interactive environment using networks Convolutional Neural (customized CNN) and VGG-16 deep transfer learning procedures on the artificial image dataset generated by CGAN, achieved test accuracy up to 55. 96% Other performance metrics for medical images such as sensitivity and accuracy achieved a score of 74. 98% and 35. 94% respectively.
This message is intended
To reduce pressure on the health system during the spread of pandemics and highly contagious diseases by relying on fast and safe methods of collecting patient data and designing accurate medical diagnostic systems.