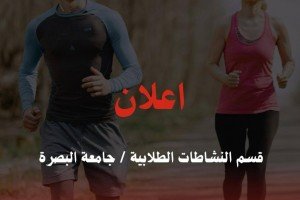
The College of Education for Pure Sciences at the University of Basrah discussed a master's thesis on improving semantic image segmentation using the U-Net model.
The message presented by the researcher (Sarah Kamel Hussein) included
Semantic segmentation of aerial and city images has gradually become a hot issue in computer vision, meaning assigning a label to each pixel in the area to give semantics to all areas of the image. However, the division of multiple categories such as buildings, roads, plants, and vehicles remains a difficult problem. Due to the overlap of the building, the roads to each other, the weather conditions, the different scales, and the high resolution of the images, all of these issues pose significant challenges.
To address these issues of poor accuracy and multiple metrics in different classes, we proposed a two-step method. The first step is to use a U-Net model which consists of a contraction pathway encoder, an expansion pathway (decoder) with skip connections generated across the decoder block in a U shape. The contraction pathway encoder forms the left side of the representation image, and the size of picture while the number of channels increases. This is due to downsampling, convolution, and the extreme assembly process. In the decoder path, on the right side of the representation image, the number of channels decreases while the image size increases due to the processes of downsampling and convolution. The second step is to use conditional random fields (CRFs)
The goal of the message:
To improve the accuracy of semantic segmentation of two databases, which serves as a post-processing, is performed on the labels of classes that are considered independent labels.High results were obtained by comparing the results with the results of previous research.