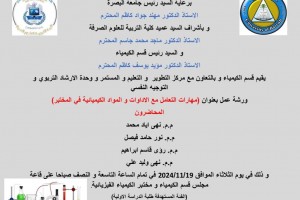
The College of Education for Pure Sciences in the Department of Computer Science at the University of Basra discussed a master's thesis entitled (Feature Reduction Based on N-gram Filtering and WordNet for Text Classification).
The thesis presented by the researcher (Zainab Mahdi Muhammad Jawad) included designing a system based on reducing the dimensions that result from the increasing growth of textual data. In addition to improving classification results by merging three classifiers to obtain the best prediction.
The process of feature reduction is using two methods: the first is by finding the correlation between words that exist in a series, through the N_gram approach, which is based on three types: tri_gram (which represents three words in a sequence), bi_gram (which represents two words in a sequence) and uni_gram ( Which represents one word) by finding the highest weighted features because they give more semantic meaning than the less weighted features.
As for the second method, it unifies the synonymous words through the use of the WordNet approach that finds semantic relationships between words. The proposed system relied on finding a synonym between words, and then creates a special dictionary for each database to unify the synonyms into one word, where this dictionary is used to unify the features and thus reduces their number. The first and second methods were combined to find the best qualities.
Finally, three classifiers were used: Naïve Bayes, Support Vector Machine, and K-Nearest Neighbor, where the proposed system combines the results for each classifier using the probabilities that gained from each classifier using soft combination to find the final prediction of the classification system. The system was applied to four data sets: 20-Newsgroups and Reuters-21578 for English and Watan-2004 and Khalaf-2018 for Arabic